Introduction
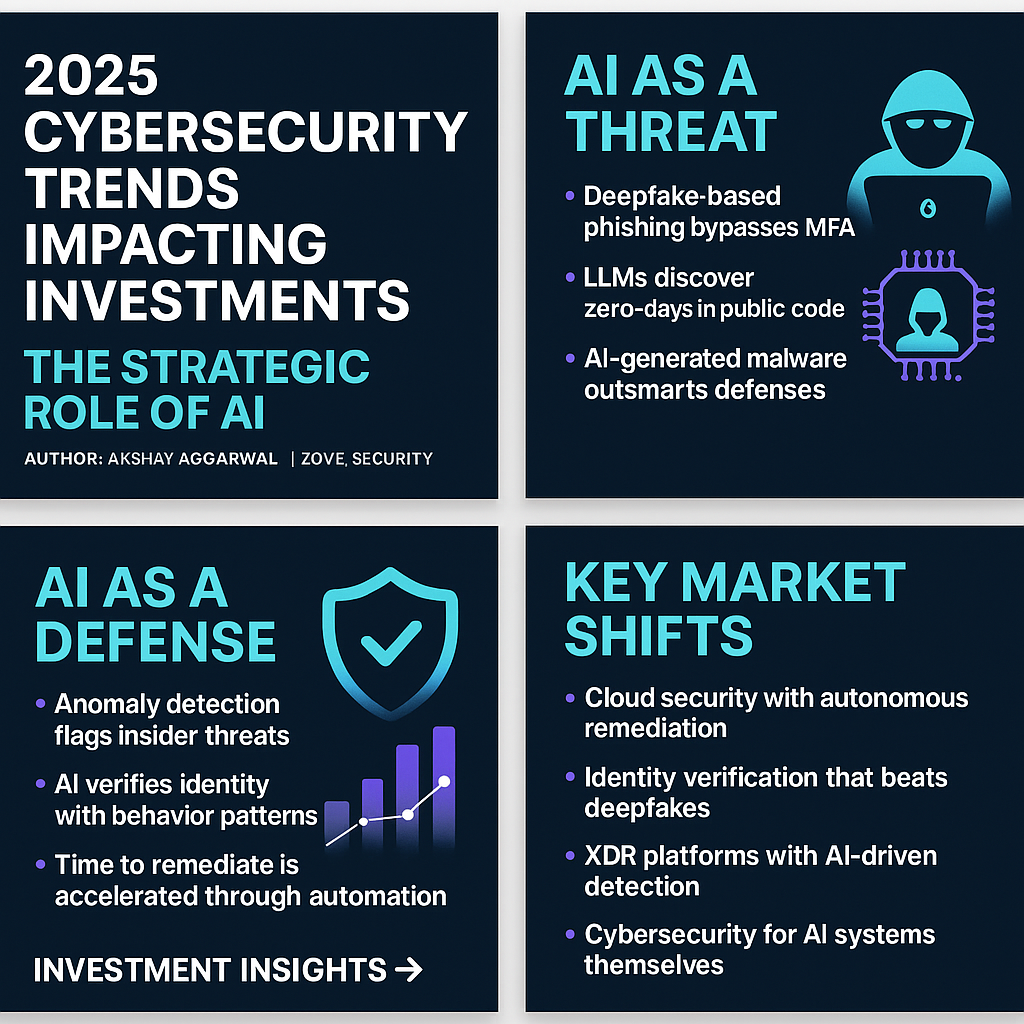
Cybersecurity is no longer a niche within software—it’s now a battleground of constant innovation, defined by adversarial dynamics and regulatory scrutiny. The defining theme of the next 24 months is artificial intelligence. AI is rapidly transforming how attackers operate and how defenders must respond. For investors, this shift creates asymmetric opportunities: firms that harness AI to automate detection, accelerate response, and protect novel attack surfaces will outpace those relying on legacy models. Conversely, AI-augmented threats will expose the limits of traditional defenses—pressuring boards, CISOs, and insurers to rethink cybersecurity spend.
I. Cybersecurity’s Strategic Differentiators from the Software Industry
The cybersecurity market is distinct from general software in ways that materially impact buying behavior and investment strategy:
- Adversary-Driven Innovation: Security solutions face an intelligent, adaptive opponent. This creates a Darwinian cycle of rapid obsolescence and product refreshes—far faster than typical enterprise software.
- Invisible ROI: Unlike CRM or ERP, the value of cybersecurity is realized by what doesn’t happen (breaches, ransom, legal fines). Buyers trust reputation, not just features.
- Regulation as a Revenue Driver: Mandates like NIS2 (EU), SEC cyber-disclosure rules (US), and upcoming AI safety standards are effectively mandating spend.
- M&A as a Constant: Acquisitions drive both innovation and exit velocity. Cyber is one of the few sectors where “acqui-hires” remain viable due to talent scarcity.
- Reliance on Platforms + Best-of-Breed Point Solutions: The vendor landscape remains fragmented despite consolidation trends, creating opportunities for both narrow innovators and platform roll-ups.
II. AI’s Emerging Role in Cybersecurity
A. AI as a Threat: Offensive Capabilities
Adversaries are already operationalizing AI to scale and sophisticate attacks. This is not hypothetical—it’s happening now, and it’s altering the economics of cybercrime.
Offensive AI Use | Description | Investment Implication |
Phishing 2.0 | Generative AI crafts hyper-personalized emails, voice and video deepfakes. | Demand spike for identity verification, behavioral biometrics, and email security. |
Vulnerability Discovery | LLMs analyze public code repos and binaries to identify zero-days at scale. | Application security and SBOM tooling (e.g., supply chain protection) are now critical. |
Malware Mutagenesis | AI generates polymorphic malware that evades signature-based tools. | Increases demand for behavior-based endpoint protection (EDR/XDR). |
Chatbot Hijacking | Prompt injection and jailbreaking attacks target AI systems themselves. | New submarket for “AI system security” is emerging—early-stage opportunity. |
The adversary’s cost to attack has dropped. Enterprises’ cost to defend is rising. This asymmetry means demand for automation, prevention, and recovery tooling will continue to outpace broader IT spend.
B. AI as a Defense: Realizable Value in 24 Months
The AI arms race isn’t just about attacks—defenders are responding. Several capabilities are already producing measurable ROI and competitive differentiation:
Defensive AI Capability | Description | Near-Term ROI (0–24m) |
Anomaly Detection (UEBA) | Behavioral analytics using ML models to spot insider threats or account takeovers. | Already embedded in major SIEM/XDR solutions. Improves detection, reduces alert fatigue. |
Automated Triage & Response (SOAR) | AI-powered playbooks reduce MTTR (Mean Time to Respond). | Cuts staffing costs and speeds up remediation. Mature in MDR/MSSP offerings. |
Threat Intelligence Correlation | ML links threat signals across telemetry (network, endpoint, identity). | Enhances efficacy of threat hunting. Drives consolidation into unified platforms. |
Generative SecOps | LLMs assist analysts by summarizing threats, suggesting queries, and writing playbooks. | Emerging, but early deployments show 20–30% productivity gains in SOCs. |
Secure Code Generation | AI-enhanced IDEs spot security bugs or generate safer code. | GitHub Copilot, Replit, and Snyk already integrating. Popular with devs. |
Defensive AI is already monetizing. Leading vendors (CrowdStrike, Palo Alto Networks, Microsoft, SentinelOne) are building moats based on proprietary threat data pipelines and ML tuning. The winners will be those who combine visibility with velocity.
III. Market Shifts Shaped by AI (2025–2027)
1. Cloud Security and AI-Native Defenses
Cloud workloads are exploding—but so are misconfigurations and lateral movement attacks. AI helps address cloud-native threats (e.g., identity drift, privilege escalation, API abuse). Expect a new wave of “autonomous cloud security” vendors or features built into CNAPPs (Cloud-Native Application Protection Platforms). Ai-enabled auto remediation from firms like HTCD will redefine and shorten window of vulnerability from months to days or even hours.
Investor Watchpoint: Companies like Wiz, Lacework, and Orca are embedding ML-based anomaly detection directly into cloud runtime. High valuation, but strong market pull. Newcomers like HTCD will fix vulnerabilities at machine scale.
2. Identity Security in the Era of Deepfakes
As generative deepfakes challenge traditional MFA and video verification, the next-gen identity market is forming around continuous authentication and passive biometrics. Expect demand for behavioral signal-based identity proofing (keystroke cadence, mouse movement, typing pressure).
Investor Watchpoint: Vendors in identity verification (e.g., AuthID, BioCatch, Ping Identity) are already pivoting toward “behavioral zero trust.” Strategic M&A targets.
3. XDR Platforms with AI-Driven Detection
Extended Detection and Response (XDR) platforms are evolving from telemetry aggregators to autonomous detection engines. The XDR of tomorrow is an AI-driven defense fabric. AI is making detection less about “rules” and more about patterns unseen by humans.
Investor Watchpoint: Leading XDR vendors (SentinelOne, CrowdStrike, Palo Alto) will either expand AI R&D or acquire to stay ahead. Look for differentiated IP in federated learning and adversarial ML.
4. Cybersecurity for AI Systems
Securing AI models themselves—preventing data poisoning, prompt injection, and model exfiltration—is now a new domain. As AI is embedded into business logic, AI security will be treated as an enterprise risk category.
Investor Watchpoint: New startups (e.g., Lakera, HiddenLayer) are emerging with niche AI security tools. It’s early but parallels the rise of AppSec 10 years ago. High-potential greenfield.
IV. Barriers to AI Adoption in Security
Despite the promise, several frictions remain for widespread AI integration:
- Explainability: CISOs are wary of “black box” AI. If a system flags a threat, they need to understand why—especially for compliance and incident response reporting.
- False Positives/Negatives: Poorly tuned models can create alert fatigue or miss subtle attacks. These damages trust in AI systems.
- Data Quality & Privacy: High-fidelity ML models require massive datasets—often containing sensitive logs. Data privacy regulations (GDPR, HIPAA) can restrict training.
- Integration Complexity: AI solutions must integrate with legacy infrastructure—SIEMs, ticketing systems, etc. Vendor lock-in and closed ecosystems are pain points.
- Skill Gaps: Operating AI-enhanced SecOps requires talent with both security and ML skills—a scarce profile.
Implication for Investors: Look for companies solving these frictions—e.g., startups offering explainable AI, synthetic data for model training, or APIs that abstract model complexity from the user.
V. Investment Implications
A. AI is an Enabler, Not a Strategy
A recurring mistake: backing a “cybersecurity + AI” pitch with no proof of problem solved. Investors should treat AI like encryption—it’s necessary, but not sufficient. The bar is real-world, referenceable deployments with measurable uplift (e.g., 30% fewer false positives, 2x faster MTTR).
B. Moats Will Be Data-Driven
The strongest AI models will be trained on proprietary, longitudinal threat data. Companies with large, diverse customer footprints and unified telemetry pipelines (e.g., Microsoft, CrowdStrike) are best positioned to compound their advantage.
C. Vertical-Specific AI Security is Coming
Sectors like healthcare, finance, and industrials will require tailored AI defense stacks due to unique data types and compliance needs. Vertical-focused security vendors (e.g., MedCrypt in healthcare) may command premium valuations as AI threats grow.
D. AI Startups Will Be Consolidation Targets
Expect ongoing M&A as legacy vendors acquire AI-native teams to stay competitive. For startups, the most likely exit remains acquisition—especially if they show technical differentiation + SOC integration readiness.
VI. Final Thought: Navigating the AI-Cyber Nexus
Cybersecurity is now a contest of data, intelligence, and speed. AI doesn’t replace defenders—but it does reshape the landscape for attackers and defenders alike. Over the next 24 months, enterprises will prioritize tools that reduce human workload, detect earlier, and automate response. Buyers will reward vendors that deliver trust through transparency and defensibility through data.
For investors, this is the moment to shift due diligence toward:
- AI capability as a product differentiator, not just a buzzword
- Explainability and integration as success indicators
- Data access and telemetry breadth as competitive moats
- Defense against both novel attacks and AI attacks
The adversary has AI. The defenders must, too. That is where the next cybersecurity alpha lies.